Video generation and multi-modality are booming this year. The impressive debut of Luma AI's video generation model Dream Machine last month has propelled the company to the forefront of this rapidly evolving space.
In previously in-depth study of Luma, which started with NeRF and focused its products and research primarily on the 3D field. This raised some important questions: What are the reasons for entering the video generation field now? What is the relationship between 3D and video generation? Will Luma's future business focus gradually shift from 3D to video, or will it pursue multiple directions simultaneously?
Overseas Unicorn invited Luma's Chief Scientist, Jiaming Song, for an in-depth interview to delve into these questions and provide an update on the company's direction a year after our first coverage. "Video is actually a path to 3D," Jiaming explained. In the process of exploring 3D, Luma completed the training of a video generation model, and the results exceeded the team's expectations.
Compared to other video generation models, Dream Machine's standout feature is its ability to generate larger-scale motions. This capability was prioritized by Luma based on user feedback. Additionally, during the training process, Dream Machine naturally developed an understanding of the physical world, demonstrating remarkable performance in understanding three-dimensional space, depth, light reflection and refraction, and how light behaves in different media and materials.
The "Bitter Lesson" is another reason why Luma is betting on multi-modal models. Language data has a higher compression ratio and yields results faster, but video and multi-modal data contain far more tokens than language, potentially leading to a more significant impact on model capabilities. Luma believes that multi-modal scaling laws will enable models to better understand the world.
During our discussion with Jiaming, we observed that although the Luma team boasts numerous researchers with impressive backgrounds, they are not solely focused on envisioning and realizing future technologies. The team's agility in product development and market responsiveness is also steadily improving. AI researchers are working diligently to bridge the gap between model capabilities and product and user needs, while the product team is focused on enhancing the model's performance from a user-centric perspective.
01 Luma's Video Generation Model: Dream Machine
Overseas Unicorn: Luma's recently launched video generation model Dream Machine has garnered significant attention. What was your role in its development? Could you tell us more about this model?
Jiaming: My primary focus in the project was on model training-related tasks, encompassing model architecture, system infrastructure, and other aspects of full-stack development. I was also responsible for coordinating the training workflow. Dream Machine is still in its relatively early stages. We opted for the DiT architecture, which is a widely accepted approach in the field.
One key differentiator we aimed for with Dream Machine was to enable it to generate larger-amplitude motions compared to existing models. We felt that users today are no longer satisfied with video models that simply create subtle movements in images without substantial large-scale motion. We wanted to push the boundaries of motion generation. While this introduces challenges related to controllability and potential imperfections, we believe that enabling the model to generate more dynamic movements is crucial for a compelling user experience. Addressing the remaining issues likely hinges on scaling up data and model size.
Overseas Unicorn: Was the decision to prioritize larger-amplitude motion driven by product insights or a technical challenge you were aiming to overcome?
Jiaming: It stemmed primarily from considerations related to product demand. We could have chosen to prioritize either smaller or larger motions. Focusing on smaller movements might have resulted in a model more akin to a text-to-image model, so it ultimately involved a trade-off. From an algorithmic design perspective, there's no particularly unique aspect to it. The key factor was determining "what kind of model do we want to present."
Overseas Unicorn: What aspects of the model are most critical when aiming for larger-amplitude motions?
Jiaming: It's primarily driven by model scale and data volume. Previous models were limited by their size and the amount of training data, making it difficult to achieve the desired outcome. Attempts to do so often resulted in unacceptable output quality. A more conservative approach adopted by others was to focus on videos with slower movements and minimal camera angle changes. As model and data scale expands, new capabilities emerge.
Overseas Unicorn: How does Dream Machine compare to other video generation models like Sora, Pika, and Runway?
Jiaming: Our approach might differ from Pika's but is likely more aligned with Sora and Runway Gen-3, with a stronger connection to Sora. They all leverage the diffusion transformer architecture.
Sora's emergence could be likened to the "ChatGPT Moment" for video models. It demonstrated the potential of this approach to deliver remarkable results with sufficient effort, prompting widespread action in the field. This mirrors the trajectory of language models. Prior to this, there were debates about Bert and GPT, but now, although research on underlying architectures for language models continues, there's a clear convergence towards the transformer architecture. I believe video models will follow a similar evolutionary path.
Overseas Unicorn: Following Dream Machine's release, what use cases have you observed gaining the most traction? In the long term, what value do you hope to provide to users?
Jiaming: Currently, our primary product format is B2C, driven by demand considerations. We aim to make this product accessible to a wider audience. We've also received numerous requests for APIs, suggesting potential for B2B applications. It's challenging to predict the specific product format for the future as it heavily depends on the actual capabilities of the model and market feedback.
02 Video is a Better Path to 3D
Overseas Unicorn: Luma's previous focus was on 3D reconstruction and generation. What prompted the shift to video generation? Luma's generated videos exhibit a good sense of space and realistic physical presentation. Is this a result of the experience gained from working with 3D?
Jiaming: It might actually be the other way around. We chose to pursue video generation as a means to achieve better 4D representation.
During our exploration of 3D, we realized that some 3D solutions faced significant hurdles in transitioning to 4D (incorporating the time dimension). The more viable approach for 3D generation currently is to train a base model using large-scale image datasets, then fine-tune it to create a multi-view 3D model, and finally transform it into a realistic 3D scene.
If the ultimate goal is to achieve 4D, there are two potential routes. The first, as I mentioned, involves using images to generate 3D and then transforming 3D animation into 4D. The alternative is to directly create a video model and then convert it into 4D. We believe the second option is more feasible. Therefore, even if we disregard the inherent benefits of video generation, simply advancing further in the 3D realm necessitates venturing into video.
Following this line of thought, we had previously fine-tuned video models for specific 3D scenes. Consequently, prior to Dream Machine, we had already established a workflow for converting video to 3D.
Another compelling reason is the relative scarcity of 3D data compared to image and video datasets. This necessitates relying on large models driven by more abundant data sources.
Therefore, our initial motivation was not to pivot from 3D to video but rather to leverage video as a catalyst for achieving superior 3D representations.
Overseas Unicorn: Could you elaborate on using video to drive better 3D?
Jiaming: We've been conducting experiments with video models for some time. Initially, we didn't anticipate significant breakthroughs in the model's ability to generate 3D content. However, we discovered that the video model's capacity for 3D generation was already remarkably robust, exceeding our expectations. The key takeaway is that the inherent 3D consistency of this video model, along with its adherence to principles of optics relevant to the graphics pipeline, is quite impressive. Naturally, it's not flawless and can falter in scenarios like gymnastics. However, its current capabilities are still remarkable. Coming from a 3D background, we're particularly attentive to 3D-related features and have observed that video can effectively handle aspects like 3D consistency, optics, depth, and dynamic physical phenomena.
For instance, we have an example where we input an image into Dream Machine, directly converted it into a video, and then fed that video into a pre-existing video-to-3D workflow. This process enabled direct interaction with the 3D model, yielding impressive results. While this might not be the ideal pipeline, it demonstrates the potential of this approach, reinforcing the notion that video could be a superior path to achieving high-quality 3D representations.
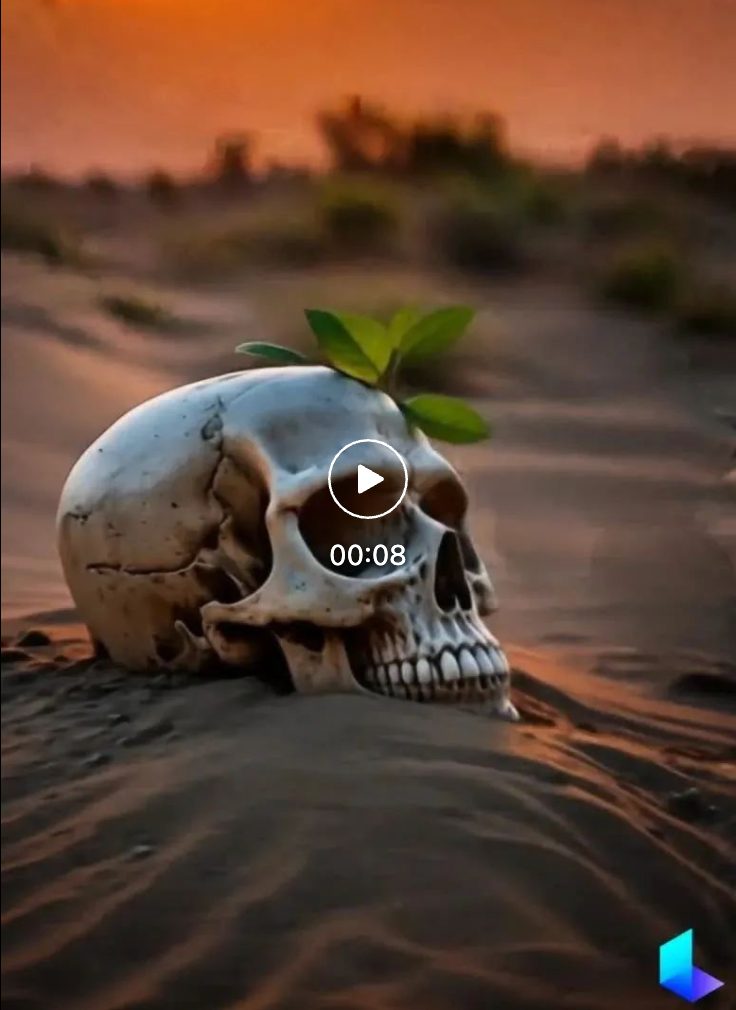
The 3D case highlights a technically intriguing point. While it's not always successful, when it works, the results are astonishing. Failures can sometimes be attributed to the lack of strict assumptions regarding the focal length and state of the video lens. While this workflow may not consistently yield positive outcomes, its ability to achieve these results underscores a notable advantage of video models over traditional solutions.
Overseas Unicorn: Can you delve deeper into these impressive effects observed in the generated videos and provide more detailed examples?
Jiaming: Firstly, simply by analyzing the video data, the model can acquire an understanding of depth. Depth, referring to predicting the distance of an object from the lens, has been a focal point in the 3D field. Even during the text-to-image era, attempts were made to address this challenge. For instance, the well-known MVDream paper utilized a text-to-image model as a starting point and fine-tuned it with depth data, demonstrating that the model could achieve good performance without requiring extensive depth data.
Contemporary video models don't necessitate special processing or the addition of depth or other 3D-related data. They can inherently learn depth information and comprehend the relative distances of objects in a video simply by processing video data. This signifies an emergent understanding of depth. Notably, this capability extends beyond realistic images to abstract imagery like Picasso-style paintings.
Below is an example of inputting a highly abstract carousel image into the video model. The model-generated video successfully simulates the carousel's rotation, indicating that even with abstract representations, the model can discern depth information.

Secondly, beyond depth, the video model can grasp concepts like light reflection, refraction, and the behavior of light in different media. Let's look at a few examples:
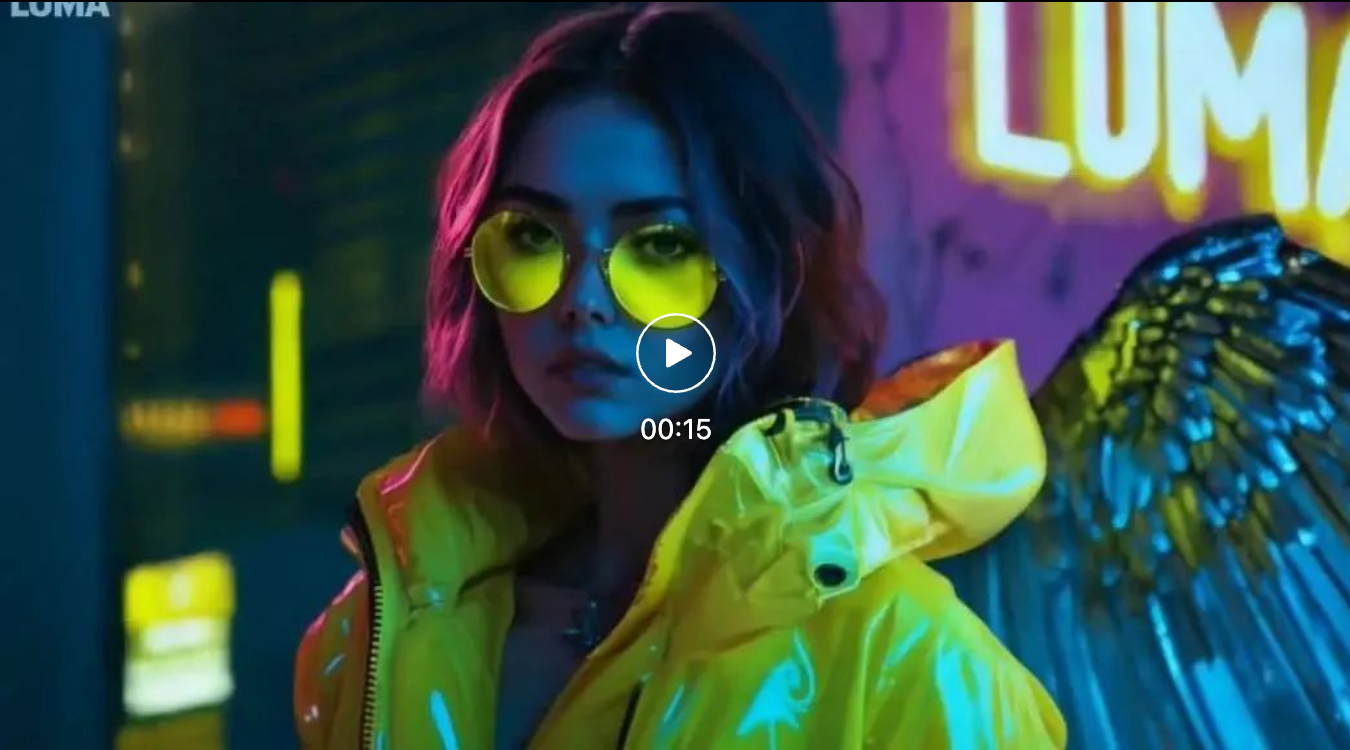
This video features a red neon light in the background. As the camera moves, the depth of the color and the area covered by light and shadow behind the person change accordingly. Simultaneously, the distance between the person's glasses and their face, as well as the thickness of the lenses, adjust dynamically with camera movement. Furthermore, simulating plastic materials based on light reflection has traditionally been challenging, but the effect achieved in this video, including its consistency, is quite remarkable.
In this coffee machine example, we conducted a comparison between traditional NeRF and video generation. Using traditional NeRF requires capturing hundreds of images around the coffee machine to achieve a satisfactory 3D reconstruction. However, with today's video models, inputting a single image of the same coffee machine allows for a faithful simulation of the coffee machine's metallic material, including the reflection of light on its surface. This demonstrates a workflow transitioning from image to video to 3D, significantly more streamlined than the previous method of capturing numerous images and reconstructing using NeRF.
This example showcases the maintenance of object consistency in high-speed, dynamic scenes. The simulation of camera movement and transitions in such high-speed environments is also quite impressive. While the simulation of distant objects or roads is not yet perfect, I believe it can be addressed by scaling up the model size.
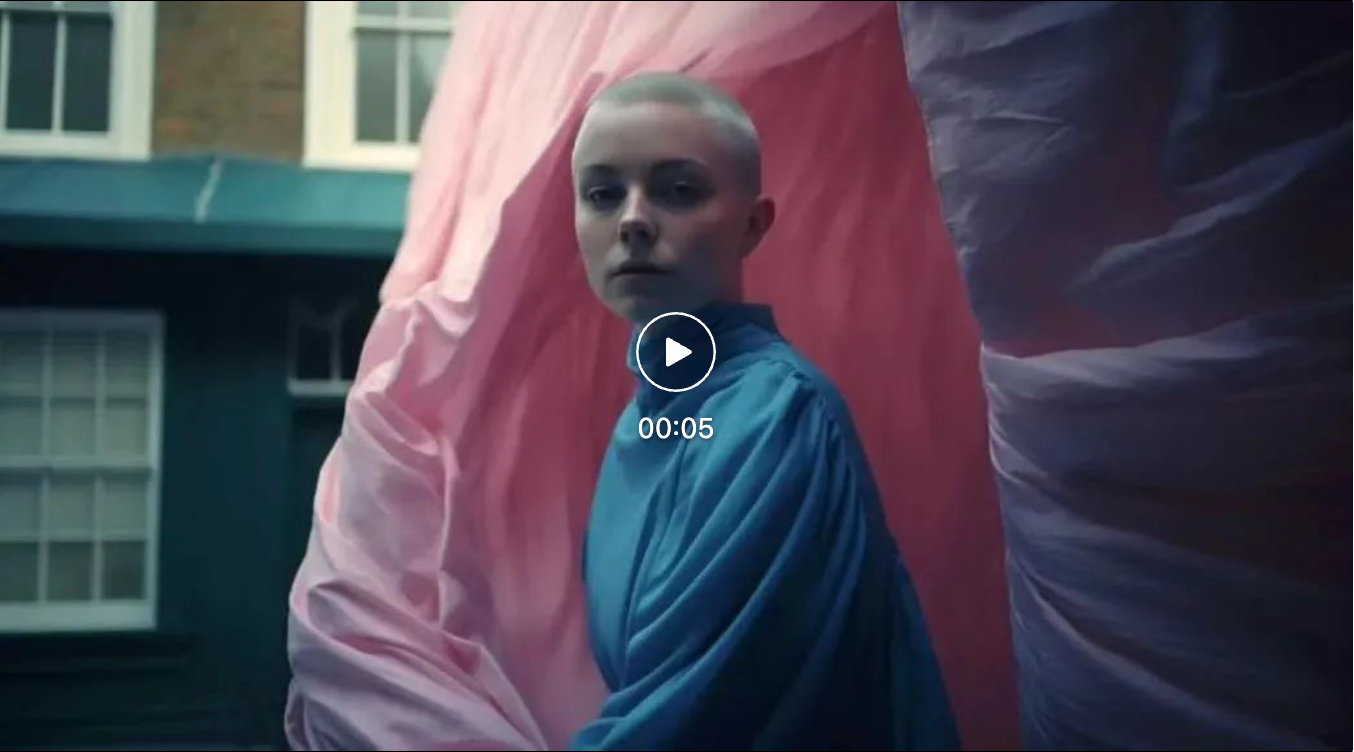
The core focus of this case is the simulation of fabric in the background. Fabric simulation in traditional game pipelines is computationally demanding, often leading to compromises in complexity. However, video models can simulate it realistically and with high fidelity at a relatively low computational cost. The texture of the fabric, along with the lighting and shadows, is simulated remarkably well.
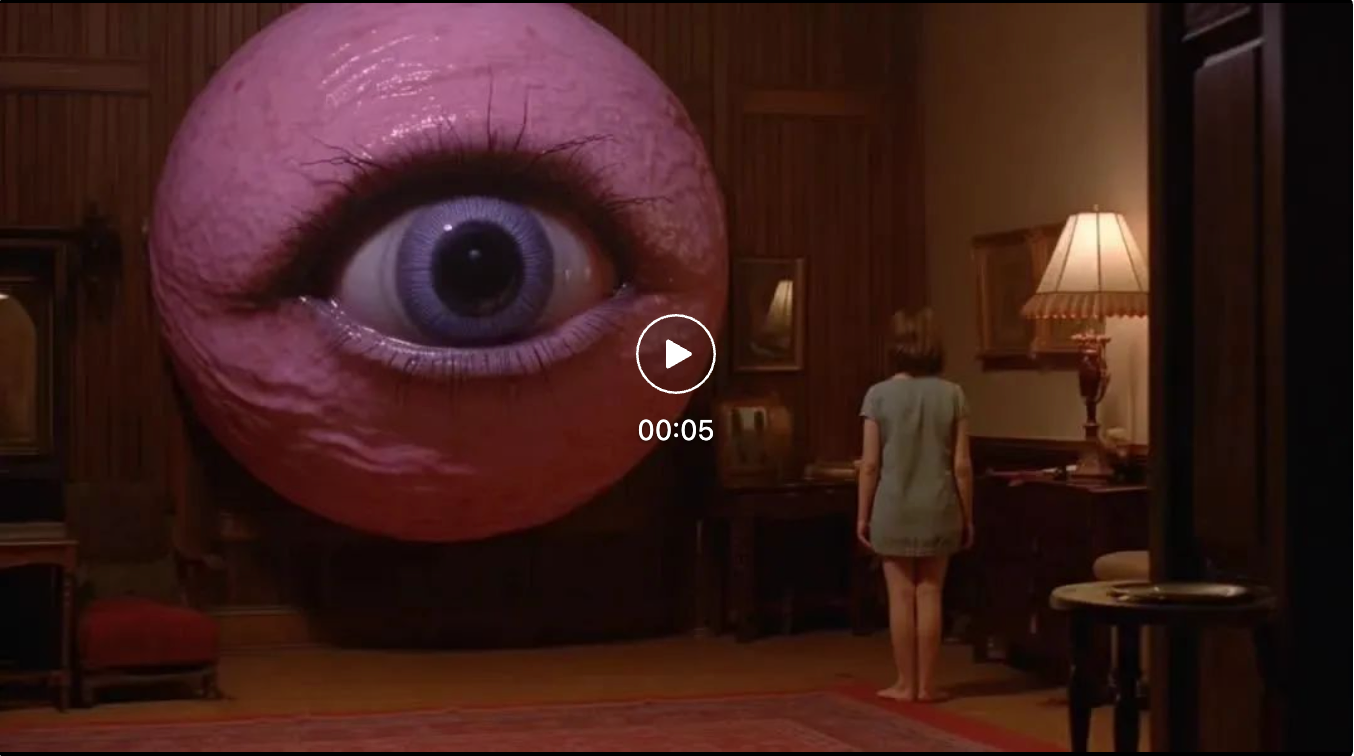
This example highlights the correlation between different scenes. Observe the young girl's frightened expression, a reaction to a scary sight in the preceding frame. The girl's hairstyle, dress color, and style remain consistent across both shots, demonstrating the model's ability to maintain correlation. This indicates that even within a continuous space, the model can effectively simulate discontinuous images and scenes.
In addition to the image-to-video cases presented above, Dream Machine's generation capabilities do have limitations.
The example appears normal for the first second. However, in the next second, a large rock abruptly appears in the background, accompanied by lightning. This clearly defies the laws of physics.
The "multi-head" problem, a phenomenon also common in 3D generation, represents another limitation of the model. This issue frequently arose when generating 3D content using text-to-image models, especially in scenarios involving rapid movements. In Greek mythology, Janus is a god depicted with multiple faces, hence the term "Janus problem" for the multi-head phenomenon.
Finally, I'd like to touch on a potential approach for achieving 4D representation through video models. A straightforward idea is to collect multi-view video data. This data could potentially be used to refine the current video model into a multi-view variant. Subsequently, the output from this multi-view video model could be utilized to generate 4D events.
Overseas Unicorn: Thank you for the demonstrations. The quality of the videos generated by Dream Machine is remarkable, particularly in terms of clarity, motion amplitude, depth of field, and lighting effects.
Jiaming: I have other noteworthy examples that I haven't included because all the examples shown here are user-generated and require attribution. We need to obtain permission from the creators before showcasing them. Unfortunately, some exceptional examples couldn't be included due to difficulties in contacting the authors.
Overseas Unicorn: Is there an evaluation framework for assessing the quality of video generation? Can specific aspects be targeted for improvement?
Jiaming: Currently, evaluation methods rely heavily on human judgment. Improvements are certainly possible, as Dream Machine is still in its early stages and has room for iteration. These improvements can be approached from various angles. For instance, if data is identified as a bottleneck, then efforts should focus on improving data quality. If the model's efficiency is the primary concern, then enhancing the model architecture becomes paramount.
Overseas Unicorn: In these examples, did the model's understanding of the physical world, particularly its grasp of camera perspective, emerge naturally, or did you dedicate specific efforts to achieve this?
Jiaming: We didn't implement any specific measures to achieve this, which makes it all the more remarkable.
Overseas Unicorn: With the rise of video generation, discussions about concepts like World Model and World Simulator have gained momentum. During the development of Dream Machine, you unexpectedly discovered its 3D capabilities. Do you envision 3D and video generation eventually replacing existing optical computing and graphics technologies?
Jiaming: They could potentially streamline certain pipelines, such as hair and fabric simulation, which are computationally intensive. However, in simpler scenarios like face rendering that don't involve hair, the necessity might not be as pronounced. Even today, hair simulation techniques are highly advanced, so the impact would depend on the specific application. Fully replacing computer graphics is still a distant prospect. However, there's potential for assisting and augmenting specific graphics pipelines.
Overseas Unicorn: For video generation models to achieve a comprehensive World Model and grasp the physical laws governing the world, will this occur as a natural consequence of model scaling, or do we need to fundamentally upgrade and transform the models themselves?
Jiaming: I believe the former is more likely. This ties directly to the concept of scaling laws, particularly what we call the "Bitter Lesson."
Richard Sutton, a pioneer in the field of reinforcement learning, proposed the Bitter Lesson. He dedicated a significant portion of his career to researching algorithms and how they can be leveraged to enhance model training efficiency. In 2019, he made a significant observation: Historically, straightforward methods that effectively utilize computational power tend to outperform approaches that incorporate extensive human prior knowledge but are computationally less efficient.
Richard Sutton cited AlphaGo as an example. Prior to the advent of deep learning, AI struggled to defeat humans in the game of Go. However, with the arrival of AlphaGo and AlphaZero, perceptions began to shift, and the Go challenge was deemed solved. One key distinction was the incorporation of more prior design, that is, algorithms based on human priors, which enabled more effective utilization of computational resources, leading to breakthroughs.
Language models exhibit a similar pattern. Significant time and effort were invested in researching language models and language understanding, tackling tasks like grammar, word segmentation, sentiment analysis, and text summarization. However, it became evident that simply by increasing computational power, language models could perform many of these tasks.
I believe video models are on a similar trajectory. Previously, each image-related learning problem required a bespoke solution based on prior experience. While these solutions could be effective, they inherently had limitations. Throughout this process, we observed that for more intricate scenarios, scaling up and adopting a more computationally intensive approach might lead to more significant breakthroughs in the long run. While it's premature to claim that video models are unequivocally superior to computer graphics, we've witnessed promising indications.
Returning to the topic of more sophisticated physical simulators, I believe the situation is analogous. As computational resources continue to advance, we might witness similar emergent phenomena in video models. Challenges that seem insurmountable today might naturally find solutions.
Overseas Unicorn: Even with model scaling, are there specific challenges that appear particularly difficult to overcome, preventing us from translating research progress into tangible results for users?
Jiaming: Numerous challenges that seem daunting now might find viable solutions as paradigms shift, approaches evolve, and computational power increases. Undoubtedly, these models have limitations, but we're still in the early stages of addressing video models, 3D representation, and controllability. There's ample room for adding new features and capabilities.
Overseas Unicorn: Over the past year, many companies, including Runway, Pika, and Kuaishou Keling, have been developing video generation models. What distinguishes Luma's approach, and what advantages do you possess in this space? Are there distinct technical paths being explored?
Jiaming: From a technical perspective, the focus moving forward should be on optimizing generation speed and efficiency.
Generation speed is linked to cost and, more importantly, user experience. I believe user experience outweighs cost considerations. From a purely financial standpoint, as long as the price of a generated video exceeds its production cost, profitability can be achieved. However, this approach might not necessarily drive significant innovation.
Imagine a video model capable of generating a five-second video in just five seconds. This breakthrough would unlock a multitude of possibilities beyond what's currently feasible. Firstly, it would enable us to cater to a far larger user base. Secondly, it would dramatically enhance the user experience. One aspect I eagerly anticipate is a significant leap in efficiency, as this would pave the way for novel product ideas.
Another frequently discussed topic is the desire for greater controllability and editability. Kuaishou Keling's example demonstrates that, even with the widespread awareness of the "ChatGPT moment" and the efficacy of scaling laws, companies in China possess remarkable capabilities in developing advanced models, boosting market confidence.
However, our target market differs from Kuaishou's. From a market perspective, there are two interpretations. One focuses on the types of products that can be created using existing models. The other, more intriguing interpretation, explores the potential for developing entirely new products based on the anticipated trajectory of model development.
Video models are currently at a stage comparable to the early days of ChatGPT in the language model domain. There's vast potential for growth ahead. Continuously enhancing model capabilities, including controllability and generation speed, is crucial, as generation speed directly impacts the business model. However, simply focusing on speed might not suffice. One could always invest in more GPUs to accelerate generation, but that wouldn't necessarily translate into cost savings. Ultimately, the optimal business model might look very different.
Moving forward, we aim to explore novel approaches and concepts that diverge from the current trends. Both model development and product development are paramount. Without a robust model, we're just an empty shell. Without a compelling product, we risk becoming a mere model-serving entity, competing solely on cost. Within Luma, our model and product teams are roughly equal in size. Our direction will be guided by user needs and our vision for future products. Some of our product ideas might seem far-fetched at present, but they will gain traction as models mature.
Overseas Unicorn: There's a perception that cost is a significant factor behind Sora's limited public availability. However, Luma's Dream Machine was publicly released and has garnered positive feedback for its user experience. How did you address the cost challenge? Do multi-modal models exhibit a predictable pattern of cost reduction and capability improvement, similar to language models?
Jiaming: It's difficult to pinpoint the exact reasons behind Sora's limited release. Cost might be a contributing factor, but they could also be carefully considering their product strategy. For instance, they might be exploring how to best integrate Sora within OpenAI's existing product ecosystem, such as incorporating it into DALL-E 3 or launching it as a standalone product.
Personally, I believe that cost reduction is inevitable. While the extent of cost reduction remains uncertain, it's bound to happen, potentially leading to new application paradigms. I firmly believe in this trajectory. Therefore, our product and model development strategies should align with this expectation. It's akin to making investment decisions; often, it's about betting on future potential rather than evaluating the present state.
Overseas Unicorn: Assuming that improvements in generation efficiency and cost reduction are inevitable, what preparations are necessary beyond simply adding more GPUs?
Jiaming: Algorithm innovation is crucial. Unlike LLMs, many areas in this domain haven't been thoroughly explored. As research progresses and new solutions emerge, algorithm innovation will undoubtedly play a pivotal role.
Overseas Unicorn: Given the exceptional performance of video models exceeding expectations, will Luma's future focus shift? How do you plan to balance the exploration of video generation and 4D directions moving forward?
Jiaming: I don't view these two as mutually exclusive pursuits. Our ultimate objective is to achieve multi-modal understanding and generation. From a data perspective, video data contains significantly more tokens compared to text data. We've previously projected that, considering both dataset size and token count, multi-modal models will be over a hundred times larger than the most extensive text pre-training models today. We've nearly exhausted native text data, prompting exploration of synthetic data generation and continuous efforts to increase model size. However, endlessly scaling model size will eventually encounter cost barriers.
From a multi-modal perspective, because multi-modal signals encompass a wealth of data, scaling laws will likely favor data-driven approaches. We might not require models as large to achieve good results.
It's worth revisiting the Bitter Lesson here. Its essence lies in minimizing reliance on human prior knowledge and maximizing the use of data and computation. To a certain extent, human language can be considered a form of prior knowledge. People speak different languages, but it doesn't hinder their understanding of the physical world and their ability to function within it.
To some degree, language itself is a compressed representation or a form of prior knowledge derived from real-world phenomena. Because language allows for greater utilization of priors, it has a high compression rate, leading to more efficient use of computation. Naturally, advancements in the language domain will occur earlier, similar to how breakthroughs in chess were achieved using search algorithms, while Go remained a challenge. It was widely believed that while progress could be made in language, achieving similar feats in video would be unlikely.
However, as data volume increases and the number of multi-modal tokens grows, the model's understanding of the world will surpass the capabilities of earlier language models. This doesn't imply that language will become obsolete, and we're not advocating for models to invent their own languages. Rather, this analogy highlights our belief in a future where multi-modality takes center stage. Whether this modality manifests as video, 4D, language, actions, or other forms is secondary.
Overseas Unicorn: The significance of multi-modal data is widely acknowledged among researchers. However, there's a concern that readily available video data, often carefully edited and entertainment-centric, might not accurately reflect the real world and human first-person perspective. This differs from language data, which is easier for AI to learn from. What are your thoughts on this? What bottlenecks might arise in the application of multi-modal data?
Jiaming: I believe it depends on the specific application scenario. Firstly, we've only scratched the surface of multi-modal data. Secondly, generating multi-modal data is relatively easier compared to text data. For instance, in autonomous driving, acquiring more data simply involves adding more cameras. Generating data using text, on the other hand, requires human effort and time. Generating low-quality data might be straightforward, but producing high-quality, fine-tuned, textbook-level data is a time-consuming process. In terms of collection efficiency, gathering text data lags behind data acquisition from cameras and other media sources.
Collecting 4D multi-view data and 3D data also presents challenges. However, new approaches might emerge, such as the image-to-video-to-3D pipeline discussed earlier, potentially addressing the data bottleneck.
Furthermore, in the 4D world, video dominates the output, and video offers greater interactivity. We might be able to utilize large models to create setups within the 4D world, but this requires models capable of faster generation and lower latency for real-time stream processing. These are exciting technical prospects.
Overseas Unicorn: Several technical approaches exist for 3D generation. Which one do you find most promising?
Jiaming: I believe the video-to-4D route holds the most promise and has a higher likelihood of success. However, we remain open to the possibility of unexplored paths leading to breakthroughs.
Overseas Unicorn: Your previous release, the Gaussian Splatting product, also garnered significant attention. You also created an interactive scene using the Gaussian approach. Will this be a focal point in your research and company strategy?
Jiaming: It depends on the progress within this specific field. While numerous papers are published at conferences like CVPR, substantial breakthroughs have been limited. One reason is the finite nature of resources, preventing us from exploring every avenue. Additionally, industry considerations differ from those in academia. For example, our Gaussian product prioritizes the quality of user-collected data. User-generated mobile phone videos often exhibit varying degrees of blur and noise, deviating from the idealized assumptions made in some academic research.
Overseas Unicorn: From an academic perspective, Gaussian Splatting has recently surpassed NeRF in popularity, with claims of superior efficiency and quality. From your product development perspective, do you share this sentiment?
Jiaming: I do believe Gaussian Splatting has its advantages. Compared to NeRF, its primary strength lies in its superior rendering capabilities on mobile devices and the web. However, it might not always adhere strictly to physical laws. It's important to note that Gaussian Splatting draws inspiration from NeRF. NeRF emerged in 2020, while Gaussian Splatting gained traction last year. In academia, it's natural to gravitate towards newer ideas.
Overseas Unicorn: You mentioned that 4D video could provide multi-angle data. Currently, both autonomous driving and robotics face challenges related to the scarcity of high-quality data. Do you believe that 4D multi-angle data could fundamentally address this issue in the future?
Jiaming: It's a possibility. However, solving this problem might not necessarily require 4D perspective data. Consider the human experience; we don't consciously inject 4D perspective data into our brains. Theoretically, humans can learn everything related to 3D through video data. Although we can comprehend 3D objects in the real world, if tasked with 3D modeling, it can still be quite challenging.
03 How Luma Defines Itself
Overseas Unicorn: There's often debate about whether a company should be research-driven or product-focused. How does Luma position itself within this spectrum?
Jiaming: We believe in embracing both aspects. We need the innovative spirit of a research lab to envision and bring to life future technologies, while also possessing the agility and user-centricity of a product company. Both are equally important and indispensable. Our researchers strive to bridge the gap between product needs and technological feasibility. Traditional academic research isn't our primary focus. However, regardless of the approach, the challenges remain similar, demanding solutions to problems that have stumped predecessors. Research prowess is paramount in this endeavor.
Luma's origins are rooted in academia, with co-founders and early team members hailing from prestigious institutions like Berkeley and Stanford. This grants us access to exceptional researchers and fosters an environment of collaboration and idea exchange. Our product team is equally exceptional, providing valuable insights to enhance our models from a user's perspective.
Overseas Unicorn: From a team composition standpoint, what's the size of the team behind Dream Machine? Was it challenging for individuals with backgrounds in 3D and NeRF to transition to this project? Did you face talent acquisition challenges?
Jiaming: Over a dozen people contributed to the Dream Machine model. I don't believe specific research backgrounds are critical; engineering skills are far more crucial. Individuals with 3D experience often possess strong engineering foundations, so we didn't encounter major hurdles in terms of team capabilities. The more significant challenge was convincing everyone to embrace video modeling. Embarking on a new and uncharted path requires rallying the team behind a shared vision. Although numerous indicators suggested this was a worthwhile pursuit, aligning everyone internally on this goal was essential.
Overseas Unicorn: This sounds more like a strategic and organizational hurdle. Within Luma, who was the first and most vocal advocate for this direction?
Jiaming: At the time, several team members recognized the potential of this endeavor. It wasn't driven by a single individual. After extensive work in 3D generation, the significance of video generation became increasingly apparent. As CTO Alex and I were deeply involved in 3D generation, we had a deeper understanding of generative models, enabling us to grasp the potential of video generation more readily.
Overseas Unicorn: Luma's initial focus was on 3D. Now, with the addition of the video generation product Dream Machine, how would you define the company's identity?
Jiaming: We don't want to be pigeonholed as a 3D company, a video company, or any other narrow categorization. The field of AI is evolving at an astonishing pace. Capitalizing on its full potential requires a multi-faceted approach.
Compared to language-based scaling, we have stronger conviction in the scaling laws of vision and multi-modality. This presents new challenges, from model and product design to exploring novel API applications.
Current research is still in its infancy. Our aspirations extend far beyond, encompassing grander visions. Product development is equally important; we can't solely focus on models. We must remain adaptable and responsive to technological advancements, shaping our company's direction based on user feedback and emerging trends. However, our core competency lies in AI, making a sudden shift to hardware development unlikely.
Overseas Unicorn: From a financial perspective, assuming a paid product strategy, do you believe the current cost structure allows for a sustainable business model?
Jiaming: Our current paid user base and ARR (Annual Recurring Revenue) are promising. However, for AI startups at our stage, achieving positive cash flow isn't a necessity, nor is it an expectation from the market. While reaching profitability is certainly feasible, it's not our top priority given our current focus. Moreover, advancements in model capabilities might necessitate adjustments to our business model. The product landscape a few months from now could look vastly different, so it's crucial to avoid being confined by our current business model.
Overseas Unicorn: We understand that OpenAI's API and ChatGPT's gross margins are not particularly high, leading to skepticism surrounding the API business model. I agree that future monetization strategies might diverge from the current norm, potentially involving tiered pricing or agent-based models where value creation dictates pricing. However, do you have a clearer vision regarding your broader business direction, specifically B2C or B2B?
Jiaming: Defining B2C or B2B models based on current models might be premature, as the arrival of a new model a few months down the line could render those models obsolete. The pace of change in business models often outpaces strategic planning. Our current priority is to relentlessly improve our models and products. This strategy is validated by OpenAI's success. Prior to ChatGPT, they lacked substantial positive cash flow. However, the launch of ChatGPT ignited widespread interest, making it significantly easier to establish viable business models. I believe multi-modal models are on a similar trajectory. Patience might be key.
Overseas Unicorn: In the future, will multi-modal generation be achievable through end-to-end models? For instance, models capable of generating images, videos, 3D content, and even audio. Furthermore, is it conceivable to combine understanding and generation within a single model?
Jiaming: I believe both are attainable.
Combining understanding and generation within a single model already has a precursor in Meta's Chameleon. Although Meta's focus was primarily on research, they didn't delve deeply into the generative aspects.
However, we need to carefully consider whether this approach is optimal from an efficiency standpoint. It might be context-dependent. Not all scenarios necessitate general-purpose models. Engineering trade-offs are often required to tailor solutions to specific needs.
Overseas Unicorn: Recent developments have highlighted Vision Pro and spatial computing. Do you see potential synergies with your 3D endeavors?
Jiaming: We've explored the possibility of developing a Vision Pro app. However, the current limited user base makes it a low-priority endeavor. We might revisit this once the market matures.
From a broader hardware perspective, the connection is stronger with 4D than 3D. Many compelling use cases, such as interactive virtual environments, rely on 4D representations. 3D might be more relevant to AR applications, but currently, compelling business opportunities in this space are limited. Achieving significant advancements in 4D could be a game-changer.
Overseas Unicorn: What are your thoughts on Fei-Fei Li's startup? They heavily emphasize "spatial intelligence," aiming to equip AI with vision and a deeper understanding of the world.
Jiaming: Their vision is intriguing, and they have a strong team with impressive credentials. One key difference I perceive is their founding team's predominantly research-oriented background, with fewer members possessing engineering and product development expertise. Only time will tell how this dynamic plays out. My personal belief is that both research and product development are crucial for success.
I'm unaware of their specific technical approach. However, when a mature and effective technical path emerges, convergence within the field is common. The widespread adoption of transformers and DiT can be attributed to their proven efficacy. While new paradigms might arise, we haven't fully exhausted the potential of existing ones. Sometimes, after a paradigm is successfully demonstrated, rapid replication and dissemination of knowledge occur within the research community. It's unlikely for a single individual or a small group to stumble upon a groundbreaking idea that others haven't considered.
Historically, it took over a year to catch up to or surpass GPT-3 with GPT-4. However, the gap between Sora, Keling, and Dream Machine was a mere four months. This suggests that if a new paradigm emerges, the speed of replication and adoption could be even faster, assuming it's a relatively straightforward solution. Replicating complex solutions would naturally be more challenging. However, based on the Bitter Lesson, the current trend favors simplicity.
Overseas Unicorn: What research direction are you most interested in now and what problem do you most want to breakthrough?
Jiaming: The direction I’m more interested in recently is more related to the system, or the paradigm of transformer itself. People now basically don’t deny that scaling law will create more intelligence, but transformer itself has a quadratic performance limitation on sequence length as the sequence gets longer. If there are more effective algorithms to solve this sequence problem, it would be a very interesting solution.
There are now some solutions based on RNN, like RNN RWKV Mamba, or some solutions based on linear transformer, but it seems that the final effect in large-scale experiments is not as good as transformer. So I think now people need to think about how to make the sequence length change from the current million level to the ten million or hundred million level while ensuring previous performance.
My own deep understanding is that any problem multiplied by 10 or 2, the solution will become very different, whether from the algorithm or from the system, it needs to be redesigned. If we can achieve a bigger breakthrough in this field, it will be very helpful for training efficiency and multi-modal understanding.
The second point I care about is the understanding of what the existing transformer or model itself is doing, or some understanding of what these models really learn, which can help us better understand or predict scaling law, enabling us to train models at lower cost or higher efficiency.
Overseas Unicorn: DiT has already used transformer, does this solve the diffusion scale problem to some extent?
Jiaming: There’s a common misunderstanding that diffusion and transformer are parallel concepts. But in fact, they are not parallel concepts, so the emergence of DiT is not saying to put two different concepts together, it’s just saying to use a method that was previously verified successful in autoregressive in the training process of diffusion.